The contribution of Generative AI in the healthcare industry will boost India’s GDP by approximately $64 billion by 2030. At the same time, AI in healthcare could become a $188 billion industry around the same time. These are staggering numbers, right? This is a minor gist of a recent report published by EY. Other top agencies, such as PwC and McKinsey, have reports that state similar statistics.
What Is Gen AI?
Gen AI or Generative AI is a subset of Artificial Intelligence (AI) that uses algorithms to generate new, original content. This could be realistic images, text, or even 3D structures based on patterns it has learned from existing data fed to it.
Unlike traditional AI systems programmed to follow predefined rules or perform specific tasks, Gen AI can process and analyze unstructured data—information that isn’t organized into a predefined format and is often challenging to interpret.
This capability is a significant breakthrough for healthcare operations that rely heavily on unstructured data like clinical notes, diagnostic images, medical charts, and audio recordings. With the global healthcare AI market projected to reach about $187.95 billion by 2030, it’s clear that AI, specifically Generative AI, is shaping the future of healthcare.
How Does Gen AI Help the Healthcare Sector?
Generative AI relies on foundational technologies like GANs (Generative Adversarial Networks) and Transformers like GPT (Generative Pre-trained Transformer) or Google’s BERT (Bidirectional Encoder Representations from Transformers).
GANs work by pitting two neural networks against each other: one generates data while the other evaluates its authenticity, resulting in more realistic outputs. On the other hand, Transformers, like OpenAI’s GPT, excel at understanding and creating text by analyzing context within datasets. These technologies have made huge strides in enabling AI to perform creative and predictive tasks that humans usually do.
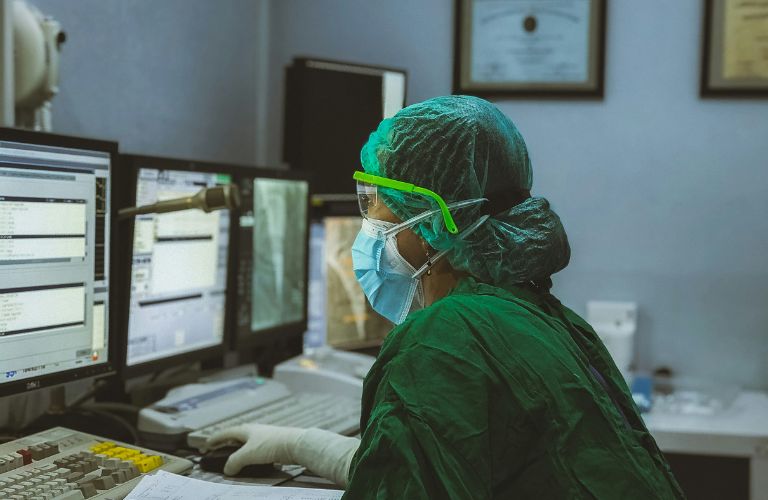
Generative AI’s ability to produce new data that mimics real-world information makes it different. In the healthcare field, these could be:
- Text: Generating coherent medical reports, research summaries, or patient education materials.
- Images: Creating synthetic yet realistic medical images for training diagnostic models.
- Molecular Structures: Designing novel drug compounds or predicting protein structures to advance drug discovery.
Generative AI’s adaptability allows it to address unique challenges. Healthcare often deals with complex, sensitive, and scarce data, such as information on rare diseases or patient populations. It can generate synthetic data that maintains the statistical integrity of real-world datasets without exposing sensitive patient information.
Additionally, Generative AI is flexible enough to adapt to numerous healthcare-specific needs, such as tailoring treatments based on genetic profiles, analyzing medical imagery to detect anomalies, and predicting patient outcomes. Its capacity to generate and refine data ensures that it can meet the ever-evolving demands of modern medicine.
What Are the Critical Applications of Gen AI in Healthcare Systems?
Generative AI is revolutionizing healthcare by introducing innovative solutions that improve efficiency, enhance accuracy, and provide superior patient care. Below are some of the most impactful applications of Generative AI in healthcare.
Enhanced Diagnostics and Imaging
One of the most critical applications of Generative AI in healthcare lies in diagnostics, particularly medical imaging.
Traditional diagnostic processes, though effective, are often time-intensive and prone to human error. Generative AI enhances these processes by analyzing complex medical images with superior speed and precision, such as CT scans, MRIs, and X-rays.
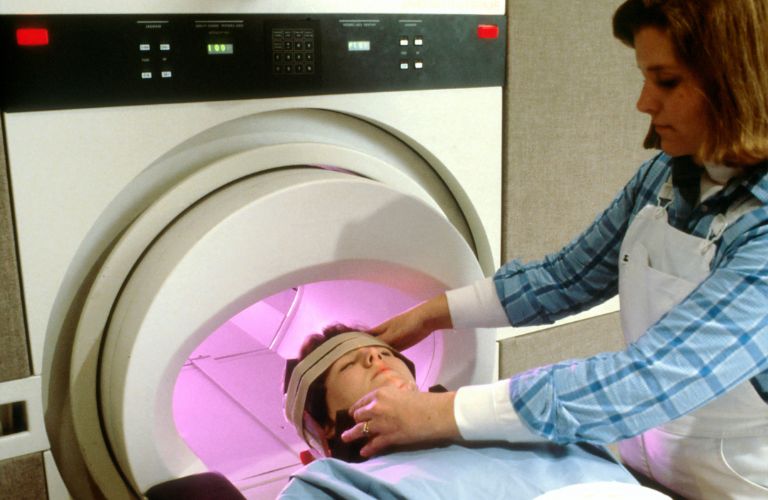
How it works: Generative AI uses deep learning models to detect anomalies, such as tumors or fractures, by comparing input images to massive datasets of previously diagnosed cases. It can identify patterns that may be too subtle for the human eye to detect, leading to earlier and more accurate diagnoses.
Generative AI can create synthetic data to train diagnostic models, particularly in cases where real-world data is scarce, like rare diseases. This synthetic data mimics real patient scenarios, enabling AI systems to learn and improve their accuracy in diagnosing rare or complex conditions.
For example, Google’s AI-powered Med-Palm 2 was trained on the MedQA dataset and achieved an 86.5% accuracy rate while answering relevant medical questions. Google admits the algorithm still needs improvement, but it’s a strong start for generative AI as a diagnostics assistant.
Personalized Medicine and Treatment Plans
Generative AI is transforming one-size-fits-all healthcare into a highly personalized experience. By analyzing patient data, including medical history, genetic information, and lifestyle factors, AI can generate tailored treatment plans optimized for individual patients.
How it works: Generative AI creates predictive models that identify the most effective treatment options based on a patient’s unique profile. It can also simulate drug interactions, reducing the likelihood of adverse effects.
An AI system could analyze a cancer patient’s genetic markers to recommend the most effective chemotherapy regimen or suggest alternative therapies if standard treatments are not advised.
[Extra read: How to Explain the Onset of Diabetes to Your Children]
Streamlined Administrative Processes
Administrative tasks in healthcare, such as medical transcription, billing, and appointment scheduling, can be time-consuming and resource-intensive. Generative AI streamlines these processes, allowing healthcare providers to focus on patient care.
AI-powered tools, such as natural language processing (NLP) systems, automate repetitive tasks by understanding and generating human-like text. They can transcribe a physician’s notes or generate insurance claim forms with minimal manual intervention. Chatbots powered by Generative AI can assist patients by scheduling appointments, answering frequently asked questions, or even providing basic pre-visit instructions.
For example, Navina, a medical AI startup, built a generative AI assistant that helps doctors tackle administrative duties more efficiently. This tool can access patient data, including EHRs (Electronic Health Records), insurance claims, and scanned documents, give status updates, recommend care options, and answer doctors’ questions. Navina has already scored $44 million in funding, which indicates a strong interest from the medical community.
Remodeling Drug Discovery
Drug development is traditionally a time-consuming and expensive process, often taking years of research and billions of dollars in investment. Generative AI accelerates this timeline by identifying potential drug candidates and simulating clinical trials.
Generative AI models analyze existing molecular data to predict new compounds or modify existing ones. It can also simulate how these compounds interact with biological systems, reducing the need for extensive physical testing.
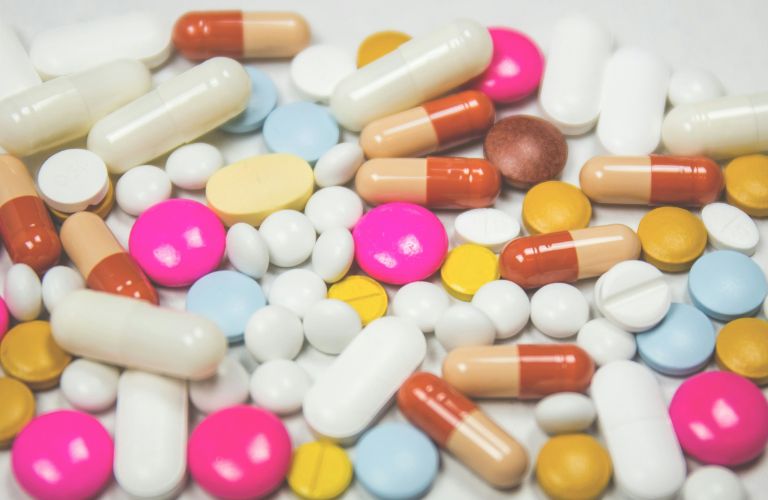
For example, in 2023, a company named Recursion Pharmaceuticals acquired two AI startups for $88 million. One of them, Valence, is known for its generative AI capabilities. It works on designing drug candidates based on small and noisy datasets that are insufficient for traditional drug discovery methods.
H2: Important Challenges and Ethical Dilemmas Posed by the Use of Gen AI in Healthcare
While Generative AI boosts healthcare ops, its adoption comes with significant challenges and ethical dilemmas. Addressing these concerns is crucial for ensuring responsible and effective use of Gen AI in healthcare.
Data Privacy Concerns
Healthcare data is one of the most sensitive information people can share, making data privacy an important concern in AI implementation. Generative AI models require a lot of data for training, including Protected Health Information (PHI) such as medical histories, diagnostic images, and genetic profiles.
Potential risk: Improper data handling can lead to breaches, exposing sensitive information to unauthorized parties. Even anonymized data used to train AI models can sometimes be re-identified through high-end techniques.
Possible solution: Organizations must implement robust data encryption, secure storage solutions, and adopt strict access controls. Using synthetic AI-generated data can mitigate risks, as it allows for training models without exposing real patient information.
Bias in AI Models
Generative AI models are only as good as the data on which they are trained. If the training data contains biases, whether due to the underrepresentation of certain demographics or historical inconsistencies in patient care, they can carry over into the AI’s recommendations or decisions.
Potential risk: Biased AI can lead to disparities in patient care, such as underdiagnosing diseases in certain populations or providing less effective treatment recommendations. This further deepens the existing inconsistencies in healthcare.
Potential solution: Having diverse and representative datasets during training is critical. Regular auditing of AI outputs for bias, combined with human oversight, can help identify and correct potential issues before they impact patient care.
Regulatory and Legal Complications
Another significant challenge is handling the complex regulatory environment for healthcare AI. Frameworks like HIPAA (Health Insurance Portability and Accountability Act), GDPR (General Data Protection Regulation), and other laws govern how medical data can be collected, stored, and shared.
Potential risk: Non-compliance with these regulations can result in hefty fines, legal action, and loss of patient trust. The last one is the most critical. Also, the lack of standardized global guidelines for Gen AI in healthcare creates confusion and slows innovation.
Possible solution: Developers and healthcare organizations must prioritize regulatory compliance by integrating approved privacy and security features into AI models. Proactively engaging with regulatory bodies to create clearer guidelines for AI applications in healthcare can also help streamline the development process.
Patient Trust and Adoption
For Gen AI to succeed in healthcare, patients must trust the technology and feel confident in its ability to improve their experience. However, skepticism around AI-driven decisions, particularly regarding diagnoses or treatment recommendations, remains an ongoing obstacle.
Potential risk: A lack of understanding about how Generative AI works, combined with fears of dehumanizing healthcare, may lead to resistance from patients and even healthcare professionals. When patients lose trust in a healthcare infrastructure, progress is very difficult.
Possible solution: Building trust requires clear communication about the role of AI in patient care. Healthcare providers should emphasize that AI is a tool to support, not replace, human expertise. Being upfront and transparent about how AI systems arrive at their recommendations can also help in handling patient concerns.
How Does the Future of Generative AI in Healthcare Look?
The next wave of innovations powered by Generative AI will likely redefine medical practices and patient care. Below are a few predictions for AI-powered innovations in the healthcare domain.
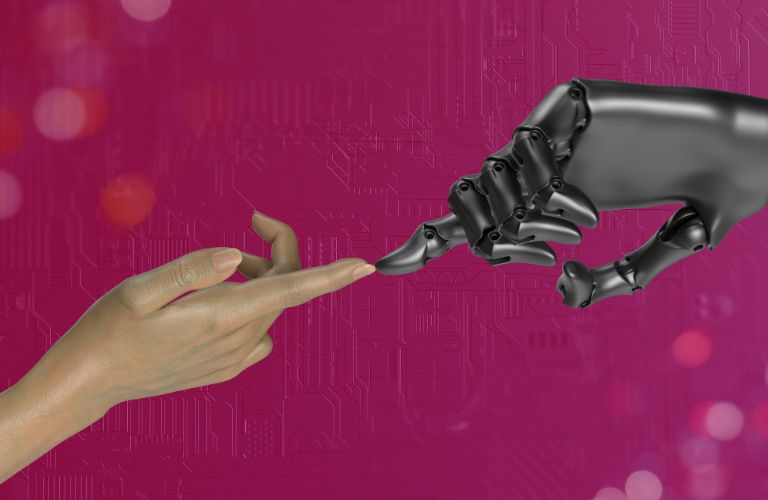
AI-powered Robotic Surgeries: Generative AI could be integrated into robotic surgical systems to improve precision and efficiency during operations. These systems may use real-time data to adapt to unexpected scenarios, minimizing the risk of complications.
Real-time Diagnosis from Wearables: The increased use of wearable health devices, like smartwatches and fitness trackers, combined with Generative AI, will enable continuous health monitoring and real-time diagnosis. AI will analyze data from these devices to predict potential health issues before they become serious.
Virtual Twins for Personalized Care: Generative AI could create virtual models, or “digital twins,” of patients. This will allow healthcare providers to simulate treatments and predict outcomes without working on real-life patients.
The future of Generative AI in healthcare is bright, offering a vision of a world where care is more accurate, efficient, affordable, and accessible. As AI continues to evolve, its ability to support innovation, improve patient outcomes, and address systemic inconsistencies will grow stronger. However, achieving this future will require a collective effort to handle challenges, build trust, and ensure that Generative AI serves the common good.
[Also read: What Is the Concept of Disruptive Innovation?]